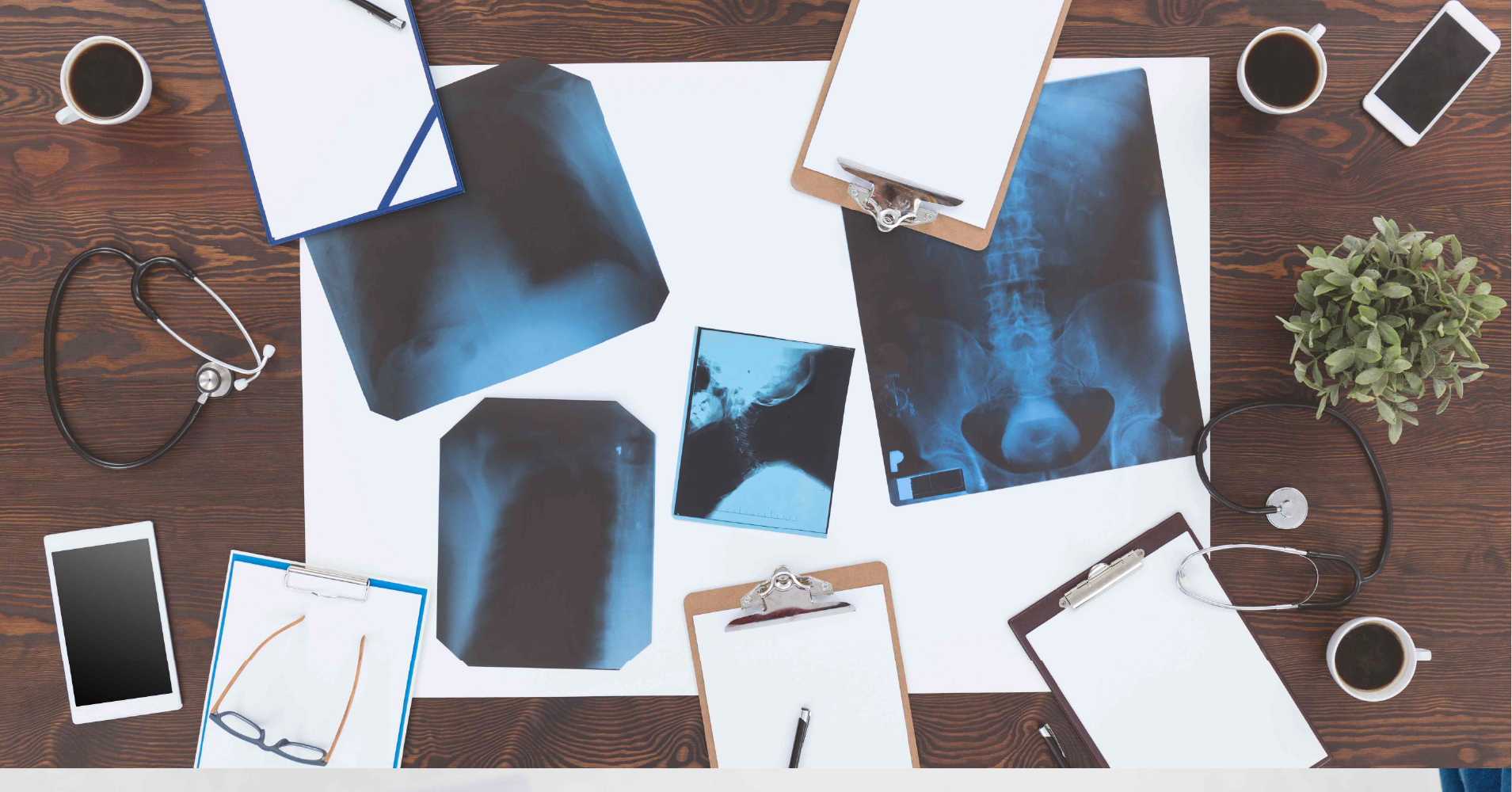
Artificial Intelligence (AI) is making significant strides in radiology, fundamentally transforming diagnostic imaging. AI is revolutionizing how radiologists diagnose and treat patients by enhancing precision, streamlining workflows, and providing innovative tools. As AI continues to evolve, its role in radiology promises to improve patient outcomes and more efficient medical practices.
Enhancing Diagnostic Accuracy
AI has the potential to improve the accuracy of diagnostic imaging greatly. AI systems can use deep learning algorithms to analyze medical images with remarkable precision, detecting patterns and anomalies that human radiologists may miss. Extensive datasets train these algorithms, enabling them to recognize subtle indicators of disease.
For example, AI can detect early signs of diseases such as cancer by identifying small tumors or abnormal tissue changes often invisible to the naked eye. Early detection is crucial for effective treatment, and AI’s ability to continuously learn and adapt from new data ensures its diagnostic accuracy will improve over time. This enhanced precision provides more reliable results, improving patient care and outcomes.
Reducing Human Error
One of the most significant benefits of AI in radiology is its potential to reduce human error. Radiologists, despite their expertise, can make mistakes due to fatigue, high workloads, or cognitive biases. AI systems, however, do not suffer from these limitations and can maintain consistent performance across numerous imaging studies.
AI can serve as a second opinion, flagging potential issues that require further investigation. This double-check system helps ensure that critical details are not overlooked, reducing the likelihood of diagnostic errors. By providing an additional layer of scrutiny, AI enhances the overall reliability of radiological diagnosis, leading to improved patient care.
Streamlining Radiological Workflows
Integrating AI into radiological workflows can significantly enhance efficiency. Radiologists often face substantial workloads, with the volume of imaging studies steadily increasing. AI can automate many routine tasks, such as image preprocessing, segmentation, and initial analysis, allowing radiologists to focus on more complex cases that require their expertise.
AI systems can also prioritize imaging studies based on the urgency of findings, ensuring that critical cases receive prompt attention. This optimization reduces patient wait times and enhances the overall efficiency of radiological services. By handling routine tasks, AI alleviates the workload on radiologists, reducing the risk of burnout and improving job satisfaction.
Collaboration Between AI and Radiologists
AI in radiology is designed to complement, not replace, human radiologists. Effective AI systems act as collaborative tools, providing preliminary analyses and highlighting areas of concern. Radiologists can then use their clinical judgment to interpret AI-generated insights within the context of the patient’s medical history and other diagnostic information.
Radiologists must train and familiarize themselves with AI tools to integrate these technologies effectively into their practice. Understanding AI’s capabilities and limitations, as well as how to interpret AI outputs, is crucial for making informed diagnostic decisions. Collaboration between AI developers and radiologists is key to creating user-friendly interfaces and ensuring that AI systems deliver practical, actionable insights.
Addressing Ethical and Legal Considerations
The implementation of AI in radiology raises several ethical and legal considerations. One major concern is the potential for algorithmic bias. If the training data is not diverse and representative of the entire patient population, the resulting AI models may produce biased outcomes, leading to disparities in healthcare delivery.
To address this issue, it is essential to use comprehensive and diverse datasets for training AI systems. Ensuring transparency in AI decision-making processes and regularly auditing AI systems for bias are important measures to ensure fairness and equity in AI-driven diagnostics. Additionally, establishing guidelines and policies is necessary to define the responsibilities and liabilities of AI-assisted diagnosis.
Data privacy and security are paramount concerns when using AI in radiology. AI systems require access to large amounts of medical data, raising questions about how this data is stored, shared, and protected. Ensuring compliance with regulations such as the Health Insurance Portability and Accountability Act (HIPAA) is essential to safeguard patient confidentiality.
Innovations and Future Prospects
The future of AI in radiology is marked by continuous innovation and exciting advancements. One promising development area is predictive analytics, where AI systems can forecast disease progression and treatment outcomes. This capability allows for personalized medicine, tailoring treatment plans to individual patient’s unique needs and conditions, thereby improving the quality of care.
AI is also being integrated with other emerging technologies, such as augmented reality (AR) and virtual reality (VR). These technologies offer immersive, three-dimensional visualizations of medical images, enhancing the ability of radiologists to interpret complex cases. AI-assisted AR and VR applications can be particularly useful in surgical planning and interventional radiology, providing real-time guidance and improving procedural accuracy.
Overcoming Implementation Challenges
Despite the potential benefits, the successful implementation of AI in radiology faces several challenges. Ongoing research and development are necessary to refine AI algorithms and expand their capabilities. Ensuring that AI systems are transparent and their decision-making processes are understandable to users is crucial for building trust and acceptance among medical professionals.
AI is transforming the field of radiology by enhancing diagnostic accuracy, improving efficiency, and introducing innovative tools and techniques. As AI technology continues to evolve, its integration into radiological practice will require careful consideration of ethical, legal, and practical aspects to ensure it complements human expertise and enhances patient care. The future of radiology, powered by AI, promises to deliver more accurate diagnoses, streamlined workflows, and, ultimately, better patient health outcomes.
Interdisciplinary collaboration between radiologists, AI developers, and regulatory bodies is essential for addressing the ethical, legal, and practical challenges associated with AI in radiology. Developing robust frameworks for validating and certifying AI tools can ensure their safety, efficacy, and reliability in clinical practice.