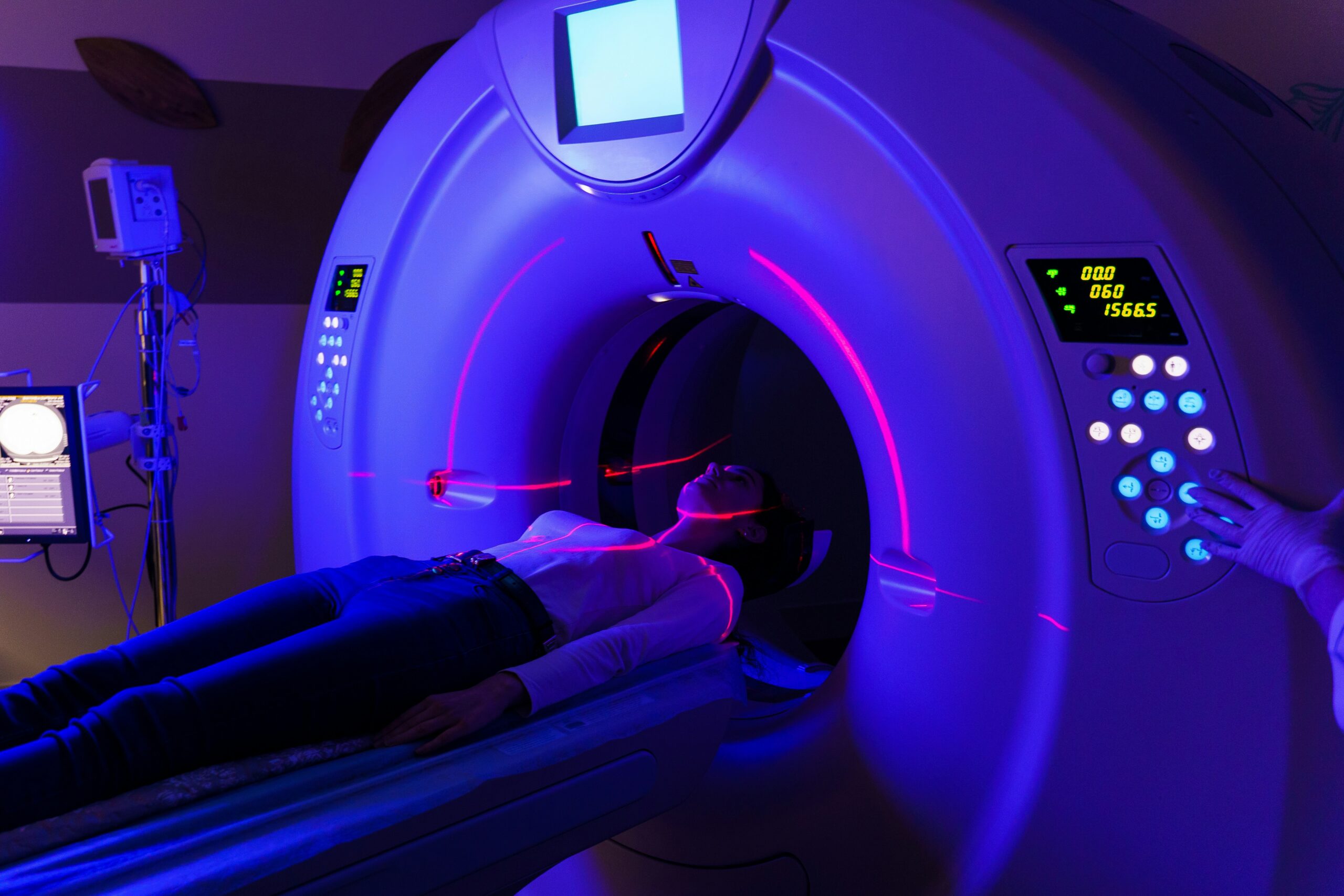
Radiology has long been a cornerstone of medical diagnostics, enabling healthcare providers to gain crucial insights into the human body through imaging technologies like X-rays, CT scans, MRIs, and ultrasounds. However, despite its pivotal role in diagnosing a range of conditions, the field of radiology is undergoing a profound transformation. This transformation is mainly driven by artificial intelligence (AI), which is reshaping how medical images are analyzed and interpreted. As AI continues to evolve, its role in enhancing imaging accuracy and improving patient outcomes is becoming increasingly evident. In this article, we will explore the exciting ways in which AI is transforming radiology, its impact on the accuracy of medical imaging, and the potential it holds for the future of healthcare.
AI-Powered Image Interpretation
One of the most significant advancements AI brings to radiology is its ability to assist radiologists in interpreting medical images with incredible speed and precision. AI algorithms, particularly those based on machine learning and deep learning, can analyze vast amounts of image data in a fraction of the time it would take a human radiologist. These algorithms are trained on large datasets, allowing them to detect patterns and identify abnormalities that may be difficult to spot with the human eye.
For instance, AI can be beneficial in identifying early-stage conditions like cancer, cardiovascular diseases, and neurological disorders. By analyzing X-rays, CT scans, and MRIs, AI algorithms can detect subtle anomalies in images, such as tumors, fractures, or blood clots; even experienced radiologists might miss that. This early detection is crucial because it allows timely intervention, potentially improving treatment outcomes and patient survival rates. As AI continues to improve, it will likely become an indispensable tool for radiologists, complementing their expertise and enhancing the overall accuracy of diagnoses.
AI-powered image interpretation increases the speed and accuracy of diagnoses and reduces the potential for human error. Radiologists are human and can experience fatigue or overlook specific details in complex images. AI, on the other hand, can consistently analyze images without fatigue, improving the overall reliability of the diagnostic process. AI can lead to more accurate and consistent results by reducing human error, ultimately enhancing patient care.
AI’s Role in Automating Routine Tasks
Another way AI enhances imaging accuracy is by automating routine tasks that traditionally require radiologists’ manual effort. For example, AI can automate image segmentation, identifying and outlining specific structures or areas within an image, such as organs, lesions, or tumors. This task, which is often time-consuming and labor-intensive, can now be performed by AI algorithms with remarkable accuracy. By automating such tasks, radiologists can focus their efforts on more complex cases that require their expertise, ultimately improving the efficiency of the radiology workflow.
AI can also help with image quality enhancement. In the past, radiologists sometimes had to deal with suboptimal images due to technical limitations or patient movement during imaging procedures. However, AI algorithms can now improve the quality of medical images by removing noise, enhancing contrast, and increasing resolution. These improvements lead to more straightforward, precise images, allowing radiologists to make better-informed decisions and provide more accurate diagnoses.
By automating routine tasks and improving image quality, AI frees radiologists to focus on critical aspects of the diagnostic process, such as interpreting complex cases and discussing treatment options with patients. This efficiency boost is significant in busy healthcare settings where time is of the essence, and radiologists are often tasked with reviewing hundreds or even thousands of images in a single day.
Integration of AI in Real-Time Imaging
AI’s integration into real-time imaging has the potential to revolutionize the way radiology is practiced. Real-time imaging refers to capturing and analyzing images immediately as they are acquired, allowing for faster diagnosis and quicker clinical decision-making. In the past, radiologists had to wait for images to be processed before they could begin analyzing them, which added delays to the diagnostic process. However, AI now enables real-time image analysis, which is especially beneficial in emergencies.
For example, in trauma cases, time is critical. AI-powered imaging systems can quickly analyze CT scans or X-rays in real-time, detecting life-threatening conditions such as internal bleeding or fractures and providing radiologists with instant feedback. This immediate analysis can significantly reduce wait times for diagnoses and allow healthcare teams to initiate treatment more swiftly. In addition, AI can provide valuable insights during procedures like surgery, helping surgeons visualize anatomical structures and track changes in real-time.
The ability to provide real-time image analysis accelerates diagnosis and improves patient outcomes by allowing for quicker intervention. This is especially important in conditions such as stroke, heart attacks, or other time-sensitive medical emergencies where every minute counts. By enabling immediate access to diagnostic information, AI empowers healthcare providers to make more informed decisions quickly, leading to better patient care.
AI-Assisted Diagnostics: A Collaborative Approach
While AI is a powerful tool, it is essential to recognize that it is not designed to replace radiologists but to collaborate with them. The future of radiology lies in integrating AI-assisted diagnostics, where AI works alongside human expertise to enhance the diagnostic process. Radiologists remain crucial to interpreting complex cases, providing context, and making clinical decisions based on their knowledge and experience. AI augments these capabilities by offering data-driven insights and improving the accuracy of image interpretation.
For example, AI can flag suspicious areas in medical images, allowing radiologists to focus on those areas. In some cases, AI can even provide a preliminary diagnosis, which the radiologist can confirm or adjust based on their findings. This collaborative approach enhances the accuracy of the diagnosis and improves workflow efficiency, as radiologists can quickly address areas that require attention rather than manually reviewing every image.
AI’s ability to collaborate with radiologists is particularly beneficial in challenging cases that require expertise from multiple specialties. AI-powered systems can analyze large datasets and incorporate patient information, such as medical history and genetic data, to provide a more comprehensive picture of the patient’s health. This data-driven approach enables radiologists to make more informed decisions, ultimately leading to more personalized and accurate patient care.
AI in Predictive Analytics: Improving Patient Outcomes
In addition to enhancing image accuracy, AI plays a significant role in predictive analytics, which involves using data to forecast the likelihood of future events, such as disease progression or treatment response. By analyzing medical images and other patient data, AI algorithms can identify patterns and trends that may indicate the early stages of the disease, allowing for earlier interventions and more proactive care.
For example, AI can analyze MRI scans of brain tissue to predict the progression of neurodegenerative diseases like Alzheimer’s, helping doctors plan treatment strategies earlier in the disease process. Similarly, AI can indicate the likelihood of cancer recurrence based on imaging data and genetic factors, allowing for tailored treatment plans that address the patient’s needs. This predictive capability improves the accuracy of diagnoses and enables healthcare providers to take a more personalized approach to patient care, improving long-term outcomes.
By integrating AI into predictive analytics, radiology can move from a reactive to a proactive healthcare model, where conditions are detected and treated before they become more serious. This shift will result in improved patient outcomes and a more efficient healthcare system.