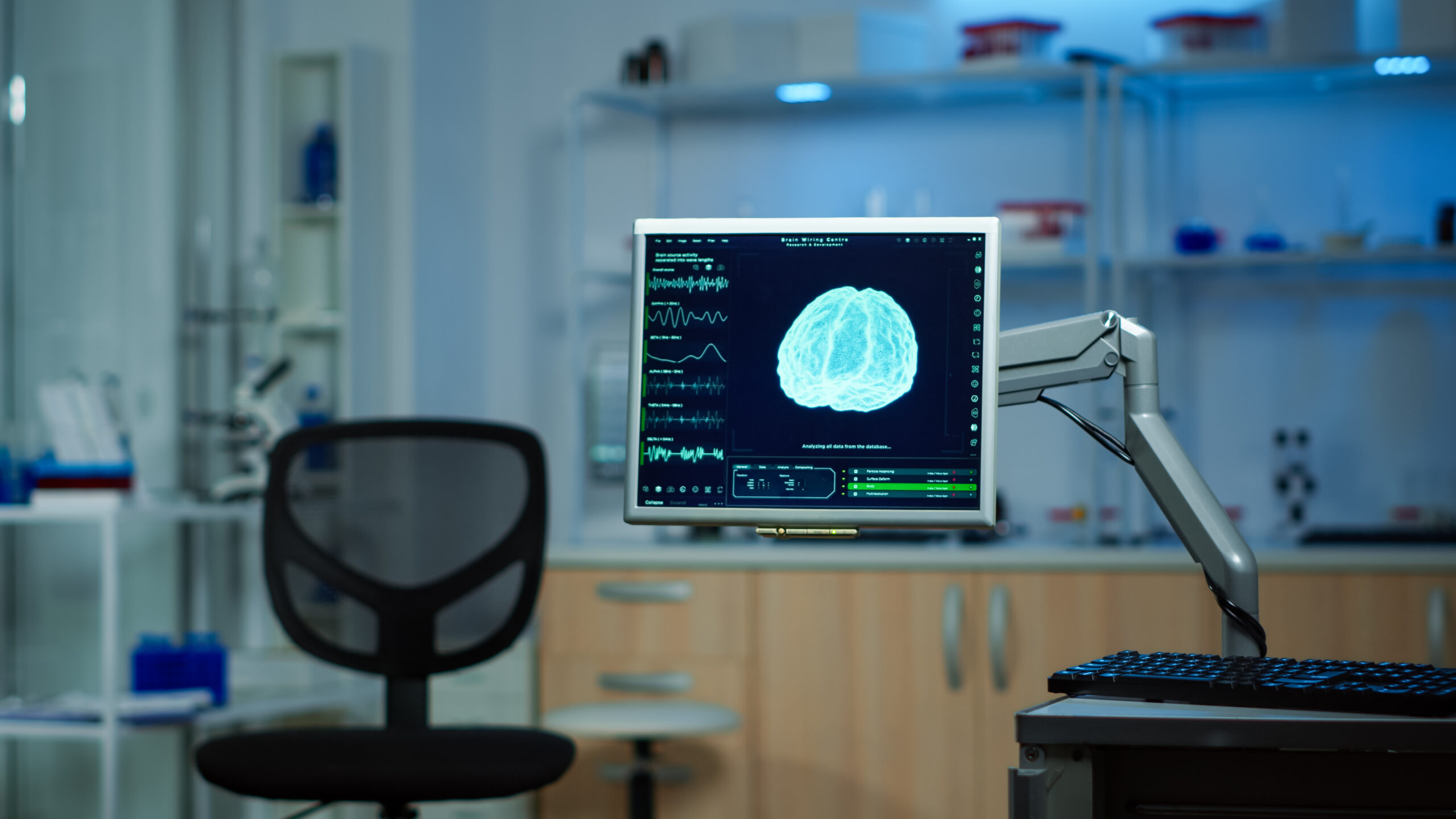
Artificial intelligence is revolutionizing nearly every aspect of healthcare, and radiology is at the forefront of this transformation. Once solely reliant on the trained eyes of radiologists to interpret complex imaging data, the field now finds itself merging traditional expertise with powerful algorithms. As medical imaging becomes more advanced, the ability to detect, diagnose, and monitor diseases accurately is evolving, thanks to AI. The future of radiology is not about replacing professionals but rather enhancing their capabilities and supporting better clinical decisions.
Transforming Diagnostic Precision with Deep Learning
Deep learning, a subset of artificial intelligence, is reshaping the diagnostic process in radiology. By analyzing vast quantities of image data, deep learning algorithms can recognize patterns and anomalies that may not be immediately apparent to the human eye. For example, AI tools are now used to identify early-stage tumors, small brain bleeds, or subtle changes in lung scans that could signal emerging health issues. These tools are trained using thousands, sometimes millions, of annotated images, which helps them refine their accuracy over time.
Several studies have shown that AI systems can achieve comparable—and occasionally superior—performance to radiologists in detecting conditions such as breast cancer, lung nodules, and diabetic retinopathy. This doesn’t mean AI is making radiologists obsolete. On the contrary, it allows them to focus on more complex tasks and reduces diagnostic errors. As AI becomes more sophisticated, the partnership between humans and machines will likely become standard practice in diagnostic imaging.
Reducing Human Error and Improving Workflow Efficiency
One of the most promising aspects of AI in radiology is its potential to reduce human error. Radiologists, after all, face high-pressure environments, reviewing hundreds of images a day. As a result, fatigue, cognitive overload, and distractions can contribute to mistakes. However, AI algorithms can serve as a second set of eyes, flagging abnormalities, prioritizing urgent cases, and ensuring consistency in image interpretation.
Beyond diagnostics, AI streamlines workflows by automating administrative tasks such as image sorting, measurements, and report generation. Radiology departments are beginning to integrate AI-driven systems that pre-analyze images before they reach the radiologist’s desk. This pre-screening reduces the time needed for a final diagnosis and ensures that critical findings are addressed promptly. As healthcare systems become more burdened by growing patient loads, the efficiency gains provided by AI will be essential in maintaining quality care. The future of radiology will likely depend on how effectively these AI technologies are integrated into clinical practice, bridging gaps in efficiency, accuracy, and accessibility.
Expanding Access to Underserved Regions
Access to quality radiological services remains a significant challenge in rural and low-resource settings. Many regions lack enough trained radiologists to meet demand, which can delay diagnoses and lead to poorer health outcomes. AI offers a scalable solution. Cloud-based platforms can deploy AI-powered diagnostic tools to remote clinics and hospitals, bringing expert-level analysis to places where specialized care is limited.
Portable X-ray or ultrasound devices with AI can quickly detect pneumonia, fractures, or pregnancy issues in the field. With minimal training, healthcare workers can capture images and rely on AI to interpret results accurately. This democratization of diagnostic expertise expands access and equips frontline providers with timely insights, marking a transformative step in the future of radiology.
Training and Adapting to the AI-Augmented Future
As AI becomes more integrated into radiology, training programs must adapt to prepare the next generation of professionals. Education must include data literacy, algorithmic biases, and the ethical implications of using AI in clinical practice.
Collaboration between medical schools, healthcare institutions, and tech companies will be vital to ensure that new tools are implemented responsibly and that clinicians remain central to patient care. Radiologists must maintain their role as decision-makers, using AI as a resource rather than a replacement. They can help shape how these technologies are used and ensure patient outcomes remain the top priority by staying informed and engaged.
Balancing Innovation with Responsibility and Regulation
With all its potential, AI in radiology raises essential questions about safety, privacy, and accountability. Regulatory bodies such as the FDA are still adapting frameworks to assess the performance and reliability of AI-driven tools. Unlike traditional medical devices, many AI systems evolve as they learn from new data, making regulation more complex.
There are also concerns about bias in AI algorithms. If the data used to train an algorithm lacks diversity, it may produce inaccurate results for specific populations. This makes transparency and thorough validation essential. Developers and healthcare providers must work together to ensure that AI applications are accurate, fair, and inclusive.
The future of radiology lies in harnessing the power of AI to enhance, not replace, human expertise. Artificial intelligence holds incredible promise, from improving diagnostic accuracy to expanding access and streamlining workflows. However, to realize its full potential, radiology must evolve thoughtfully, combining innovation with education, regulation, and a deep commitment to ethical practice. As technology advances, the partnership between radiologists and AI will become one of the most transformative alliances in modern medicine.